Spam Detection - NLP
- Rechita Singh
- Mar 3, 2024
- 1 min read
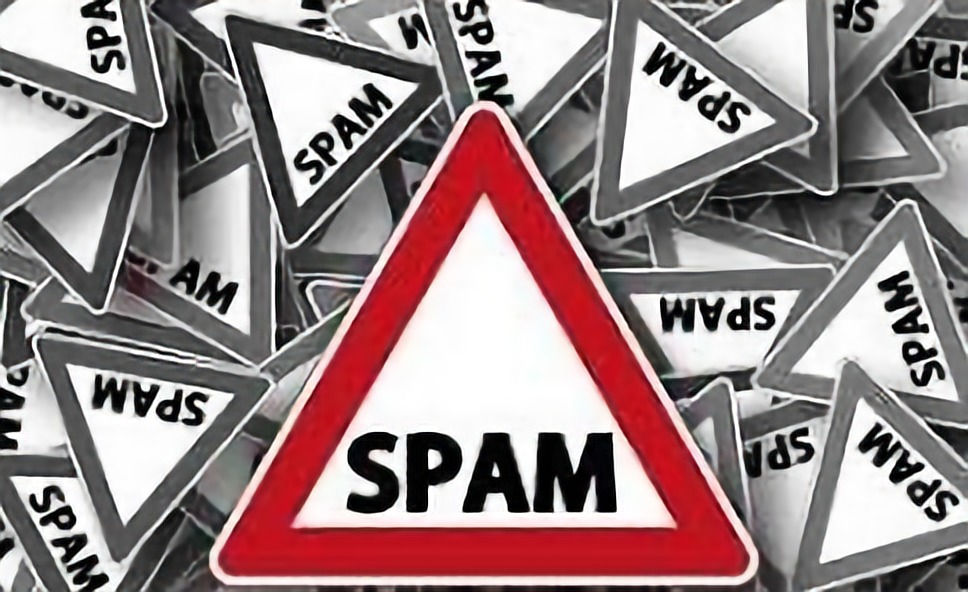
Project Highlights:
Impressive Performance: Achieved a remarkable 99% precision and 94% recall with our final model based on Pipeline3.
Methodology: Implemented a Spam Detection model using TF-IDF Vectorization and Feature Engineering techniques.
Key Steps:
Data Loading: Utilized the Kaggle spam dataset, loaded it into a Pandas DataFrame, and performed necessary preprocessing.
Evaluation Metric: Recognized the imbalanced nature of the data and carefully selected an appropriate evaluation metric to ensure meaningful results.
Classification Pipelines: Explored various feature creation methods, conducted analysis, and hyperparameter tuning to optimize model performance.
Model Performance: Achieved high precision and recall rates, with F1-scores indicating robust performance across 'ham' and 'spam' classes.
Final Takeaway:
Exceptional Model Performance: Our Spam Detection model, based on Pipeline3, demonstrated outstanding performance on the test data, highlighting the significance of choosing the right evaluation metric for imbalanced datasets.
Comments